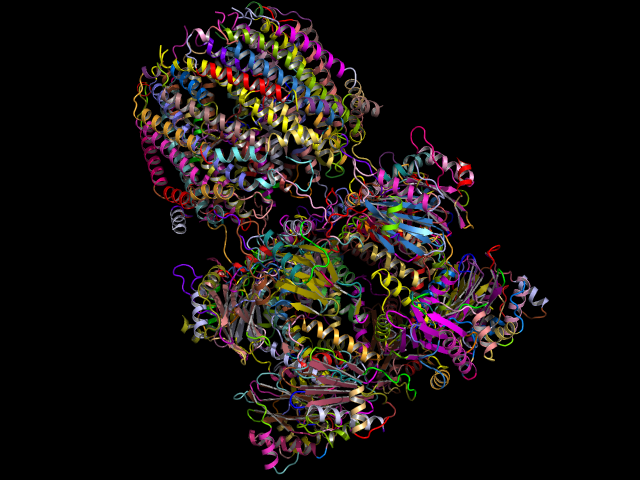
A novel approach in computational biology that previously would have taken about six years to complete, took just two weeks using RCC’s parallel computing resources.
With the help of high performance computers Tinaroo and FlashLite and RCC expertise, an Australian–US research team made significant inroads in creating a comprehensive dictionary that is able to describe effectively the known repertoire of protein structures.
The research team is deploying a unique approach to identify the recurrent structural themes that aids in understanding the general principles of protein architecture and structure.
Over the last 50 years, worldwide experimental efforts to resolve structures of proteins to atomic resolution have resulted in a rapidly growing public database, the Protein Data Bank (wwPDB). As of today, wwPDB collects more than 200,000 protein domains that fold into a wide variety of three-dimensional shapes, or folding patterns.
Understanding the principles underpinning protein-folding patterns, their architecture and structure remains central to driving advances in biology and medicine. Specifically, unraveling the protein structural determinants in terms of recurrent substructural concepts is a fundamental problem of structural biology. Such efforts help rationalise the structural descriptions of otherwise complex protein shapes.
These substructural concepts form the building blocks of protein three-dimensional shapes (analogous to LEGO bricks) — each protein in the corpus of known structures can be assembled and explained using a collection of these concepts.
The Australian-American cross-disciplinary research team, led by Monash’s Dr Arun Konagurthu, is going beyond previous investigations to discover a comprehensive dictionary of substructural concepts based on the statistical compressibility of structural data. The work is being conducted without any supervised or prior knowledge of these substructural patterns and are inferred automatically.
The innovative methods supporting the research are derived from various computer science fields, including information theory, statistical inductive inference, combinatorial optimisation, discrete algorithms, and parallel computing.
“The search problem that our unsupervised inference methodology poses is very large, complex and nuanced,” said Dr Konagurthu. “High Performance Computing expertise from RCC and its resources Tinaroo and Flashlite were indispensible to address this problem.”
Both Tinaroo and FlashLite support high performance parallel computing. The initial versions of the codes Dr Konagurthu and his colleagues used were sequential – that is, they only ran on a single processor. RCC Director Professor David Abramson worked with the team and produced a version that could utilise multiple processors, reducing the execution time dramatically.
“Using just 240 cores out of a total of over 6,000 cores on Tinaroo has delivered a speedup of over 170 times, and allowed us to infer a dictionary on a data set with over 50,000 protein domains in just 14 days,” said Dr Konagurthu.
The research team is planning a much more significant run with the entire Protein Data Bank data as input, consisting of more than 200,000 protein domains. “We expect to be able to solve the full Protein Data Bank even faster than the cut down version by applying thousands of processors, all working concurrently to solve the problem,” said Professor Abramson.
This cross-disciplinary research program was led by Dr Arun Konagurthu (Monash University) in collaboration with the following colleagues from multiple institutions:
- Prof. David Abramson (University of Queensland)
- Dr Lloyd Allison (Monash University)
- Prof. Maria Garcia de la Banda (Monash University)
- Prof. Arthur Lesk (Pennsylvania State University)
- Prof. Peter Stuckey (University of Melbourne)
- Dr Ramanan Subramanian (Monash University).
An Australian Research Council Discovery Project grant ((DP150100894) is supporting this research.