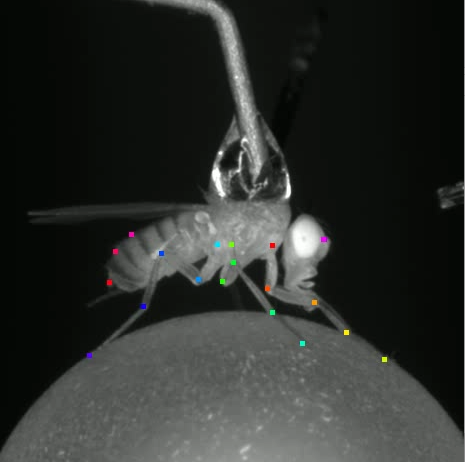
A UQ investigation into the fundamental principles of insect locomotion may one day aid the design of robots to move more efficiently.
Dr Kai Feng, a Research Fellow in the Queensland Brain Institute’s (QBI) Dickson Lab, is part of a research team using the fruit fly Drosophila melanogaster as a model organism to study the circuit mechanism of insect movement. (1)
The researchers are using deep learning in their work and UQ high-performance computer Wiener to run machine vision algorithms to make analysis of large amounts of high-speed videos of fruit flies in motion possible.
“Wiener has allowed us to train our neural network and process the videos very fast,” said Kai.
“We need to train the network, assess the quality and generate more training sets in many iterations to get satisfactory results. So, it is crucial to have fast feedback.”
Wiener, containing the most powerful graphics processing units (GPUs) ever made, is specifically for imaging-intensive research, artificial intelligence and machine learning research at UQ.
Originally inspired by discoveries in neuroscience, deep learning is in turn revolutionising the way neuroscience research is conducted. It has also transformed the way researchers study animal behaviour. (2)
“Cutting-edge machine vision methods for analysing human poses has been adapted to the laboratory to study model animals’ behaviour in unprecedented details,” said Kai.
“For a small body length and fast-moving insect like Drosophila, methods were established recently to track the precise movement of all joints on all six legs while they are walking or grooming with minimal human intervention.” (3)
Kai and his Dickson Lab colleagues have developed genetic methods to label and manipulate individual neuron types in the fly’s ventral nervous system, “the motor centre” equivalent to our spinal cord.
With the help of the new machine vision technology, the researchers can answer questions such as the exact role each neuron plays in a complex sequence of movements like backward walking or turning, and decipher the general principles of how motor programs are generated by neural circuits.
As well as improved robot design, this research may provide deeper insights into how neural circuits process information and perform complex motor tasks.
For this work, the QBI team of four researchers are collaborating with Dr Ryo Minegishi at the Janelia Research Campus, a scientific research campus of the Howard Hughes Medical Institute (HHMI) in Virginia, USA.
Professor Barry Dickson of both QBI and HHMI is leading the project.
“We have obtained a rich collection of fly strains each of which will allow us to manipulate a small subset of neurons in the motor circuits that generate locomotion patterns,” said Kai.
“Next, we want to establish a [computational] pipeline to study the behavioural change of flies in great detail when we manipulate those neurons. Basically, we will take high-speed videos from multiple views when a fly is walking on a suspended ball and analyse the joint kinematics.
“The biggest challenge here is to analyse the TBs [terabytes] of movies and generate low-dimensional representation of the fly’s behaviour.”
Before he started using Wiener, Kai worked on a machine that was one-twentieth of Wiener’s speed. “It was basically hopeless to get the [machine learning] training done,” he said.
RCC Chief Technology Officer Jake Carroll and Irek Porebski, a Senior Systems Programmer at QBI, helped Kai and the team set up the software they needed on Wiener, trained them in the optimal ways to use the HPC and other large-scale computational infrastructure RCC manages, and provide ongoing support. "They are really helpful in that they always listen to our needs and come up with good solutions,” said Kai.
Jake said: “Kai came to me initially asking about ways to accelerate and how to achieve better computational capability when his group complained about how slow training was on normal desktop or workstation GPU platforms. I then said, ‘It is time to supercompute!’”
Please contact RCC if you would like to discuss using Wiener in your research.
- https://rd.springer.com/chapter/10.1007/978-3-030-24741-6_32
- See this Nature news article as an example: https://www.nature.com/articles/d41586-019-02942-5?WT.ec_id=NATURE-20191003).
- See https://github.com/AlexEMG/DeepLabCut and https://github.com/NeLy-EPFL/DeepFly3D.
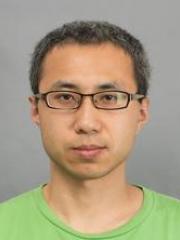