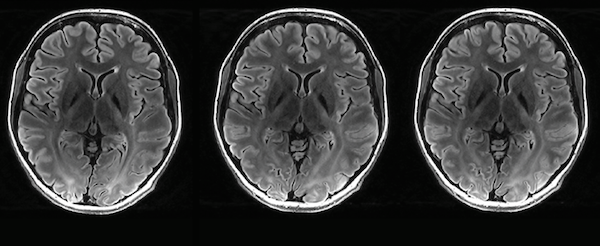
UQ researchers are using machine learning in an effort to speed up Magnetic Resonance Imaging (MRI) reconstruction and improve its affordability and pace for patients.
Dr Steffen Bollmann, a Research Fellow in UQ’s School of Information Technology and Electrical Engineering (ITEE) and an Affiliated Research Fellow in UQ’s Centre for Advanced Imaging (CAI), and a range of UQ and international collaborators are applying machine learning (ML) techniques to several image processing problems.
MRI is a powerful non-invasive imaging technique for diagnosis as well as understanding the structure and function of the brain and body.
MRI has been revolutionised by several new high-resolution imaging technologies in recent years. However, large amounts of data lead to slow processing times and in many cases manual annotation of the imaging data is both common and very time consuming.
Steffen and his fellow researchers aim to use ML to speed up MRI image reconstruction, reduce scan time and automatically identify, quantify and annotate images to bring the latest advances in MRI into clinical and neuroscience applications.
The ultimate goal is to make MRI more affordable outside of specialist imaging centres and increase patient throughput, diagnostic accuracy and patient comfort.
“Machine learning is a powerful tool to solve challenging problems in medical imaging,” said Steffen. “Translating these exciting results into robust applications that save time and cost and lead to new insights and better clinical outcomes needs to be our priority.”
The researchers have developed six different ML applications involving imaging for the human brain and body, including brain blood vessels, the brain’s hippocampus associated with memory and learning, and different types of body fat.
Dr Nick Hamilton, RCC’s Senior Machine Learning Consultant, who is helping coordinate machine learning support and training at UQ, assisted the researchers with training and was on the PhD committee for Dr Thomas Shaw, now a Postdoctoral Research Fellow in ITEE and an Affiliated Research Fellow in CAI.
“The size of the data sets and the machine learning models [the researchers used] is really pushing the limits of current computational hardware, storage hardware and software methods,” said Nick. “Many of the solutions they have developed to work with and around these limits are really interesting and no doubt many other machine learning users or providers could learn from them.”
The UQ researchers are making the most of the University’s computational infrastructure, including resources available through RCC.
Their principal platform for computation was Wiener, an RCC-managed Graphic Processing Unit (GPU) high-performance computer at UQ.
Wiener is designed to expedite the pace of research in a diverse range of imaging-intensive science generated by UQ’s world-leading microscopy facilities.
“Wiener was a game changer for our work as it enabled us to train multiple large models in parallel and test different training parameters in a short amount of time,” said Steffen.
For data storage and access, the researchers used UQ’s Research Data Manager (RDM) system based on the RCC-managed MeDiCI data fabric, which enables ultra-fast multi-site data access on demand without user involvement.
“Image data set sizes and the number of files are pushing the limits of current storage, especially when fast access is needed to train machine learning models on the data,” said Nick. “The MeDiCI storage fabric connected to the Wiener supercomputer was an enabling factor for many of the projects.”
For the hippocampus, blood vessel and body fat projects, the Characterisation Virtual Laboratory (CVL) was used to process and visualise data needed for the ML models. UQ is one of CVL’s eight partners.
“With COVID-19 restrictions, we found remote desktops such as CVL, which provide both a visualisation environment to allow annotation and correction of training data, as well as access to HPC and GPU systems, to be invaluable,” said Steffen.
To enable the specific neuroimaging data pre-processing on CVL, Steffen and his collaborators developed NeuroDesk, a flexible, scalable and easy-to-use data analysis environment for neuroimaging running on CVL. NeuroDesk is publicly available and free for researchers to use.
Oracle for Research supported the NeuroDesk project with a compute grant on its cloud infrastructure.
The researchers also used other external computational resources, such as Google’s Colab and Tensorflow, the core deep learning framework for most of the projects.
As ML is a relatively new field, upskilling researchers in its use is essential. Nick is coordinating an ML training exchange with Monash University. This enabled Thomas to attend an introductory workshop on deep learning for Tensorflow last November, and Steffen to attend a semi-supervised deep learning workshop in March this year — both hosted by Monash.
Reciprocally, Nick has developed and run new ML workshops for imaging this year, which have been open to both UQ and Monash researchers.
The training exchange is part of an ARDC-funded project bringing together ML tools, libraries, and access to data, across large GPU deployments nationally to provide a consolidated platform for ML-based research, deployed at Monash and UQ.
UQ has also been running other ML events. In January this year, ITEE held a Deep Learning Hackathon during the UQ Summer School of AI organised by Steffen and Dr Shakes Chandra, an ITEE Senior Lecturer. “This was invaluable both as a venue to rapidly develop new neuroimage analysis tools and methods, but also as a venue to share skills, expertise and experiences between the participants,” said Steffen.
Applications of ML to brain and body imaging has been a productive and successful stream of research for Steffen and his collaborators. It has led to six papers being published so far, including in the journals NeuroImage, NMR in BioMedicine and Magnetic Resonance in Medicine.
Steffen was also recently awarded an ARC Linkage grant that will advance deep learning applications for medical imaging and improve the robustness and interpretability of the predictions.
“The reviewers of the grant were particularly impressed by the computational infrastructure available at UQ, highlighting that the unique combination of compute (Wiener) and storage (MeDiCi) are crucial for the proposed project and contribute to the cost-efficiency of the proposal,” said Steffen.
“I am very much looking forward to this project — it feels like we are still in the early days of machine learning and bringing intelligent machines into medical imaging is deeply exciting.”
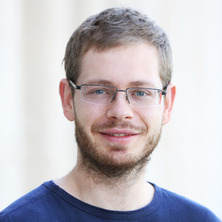