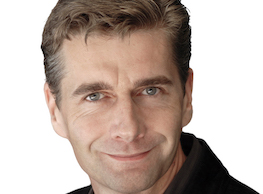
Solving advanced microscopy imaging data bottlenecks and automating skin cancer diagnoses are the topics of two recently-published papers featuring UQ and RCC Senior Machine Learning Consultant Dr Nick Hamilton as senior author.
The two peer-reviewed, journal-published papers involved machine learning and RCC-managed high-performance computers to achieve results.
Nick’s paper titled ‘LLAMA: a robust and scalable machine learning pipeline for analysis of large scale 4D microscopy data: analysis of cell ruffles and filopodia’ was published online in BMC Bioinformatics on 19 August this year.
The paper details the work and results of a three-year ARC-funded project, with Nick as the lead Chief Investigator, involving machine learning for organelle selection and feature detection in live cells.
Dr James Lefevre, Dr Yvette Koh, Dr Adam Wall, Dr Nicholas Condon and Professor Jennifer Stow—all from UQ’s Institute for Molecular Bioscience (IMB)—are also listed as co-authors of the paper.
Their project will conclude at the end of this year and has involved UQ’s Lattice Light Sheet Microscope (LLSM), installed at IMB in 2017.
“Lattice light sheet microscopy provides a window into biological function in real space and time, generating unprecedented insights into cell behaviours,” said Nick.
“LLSM has revolutionised laser imaging by allowing 4D, high-resolution, extremely rapid and long-term imaging of fluorescent probes in living cells. But it has created a major data processing bottleneck with an urgent need to develop new analytic methods tailored for extracting, quantifying and analysing data in these massive image files.”
The project developed machine learning pipelines—backed by large-scale RCC-managed HPCs Awoonga and Wiener—to automatically find, quantify, track and visualise multiple cell surface structures in high resolution as they form and evolve in real time.
“This is then applied to provide insights into fundamental cellular processes such as macropinocytosis, a pathway that cells use to internalise proteins and molecules as well as drugs, and the immune response,” said Nick.
Nick’s second paper, titled ‘Characterization of tissue types in basal cell carcinoma images via generative modeling and concept vectors’, is online and will be published in Computerized Medical Imaging’s December 2021 issue.
PhD student Simon Thomas, who works in Nick’s IMB lab, IMB’S Dr James Lefevre and Dr Glenn Baxter from Queensland’s MyLab Pathology are co-authors of the paper.
Nick was involved in the conceptualisation and supervision of the work primarily done by Simon.
In previous work, Simon has used machine learning methods and HPC Wiener to create near-perfect automated classification and distinction of three types of skin cancer pathology imaging. (See our separate case study about Simon’s research.)
In the work the recent paper is based on, Simon used generative machine learning methods to learn the structure of the features in cancer imaging.
“These can then be used to generate artificial images of skin cancer that are close to indistinguishable from the real images demonstrating that the methods ‘understand’ and ‘see’ the imaging and structure in as much as we or a trained pathologist does,” said Nick.
“In this way, rather than being a ‘black box’, as many machine learning methods are, it provides insights which are naturally interpretable. This then has applications to classify cancer types, as well as to giving much more subtle measures of the degree of the cancer through a machine learning notion known as ‘concept vectors’.”
This is the second paper produced as part of Simon's PhD project that started in 2018. He hopes to submit his thesis by the end of this year.
Simon has recently accepted a position at CSIRO in machine learning to start next year.